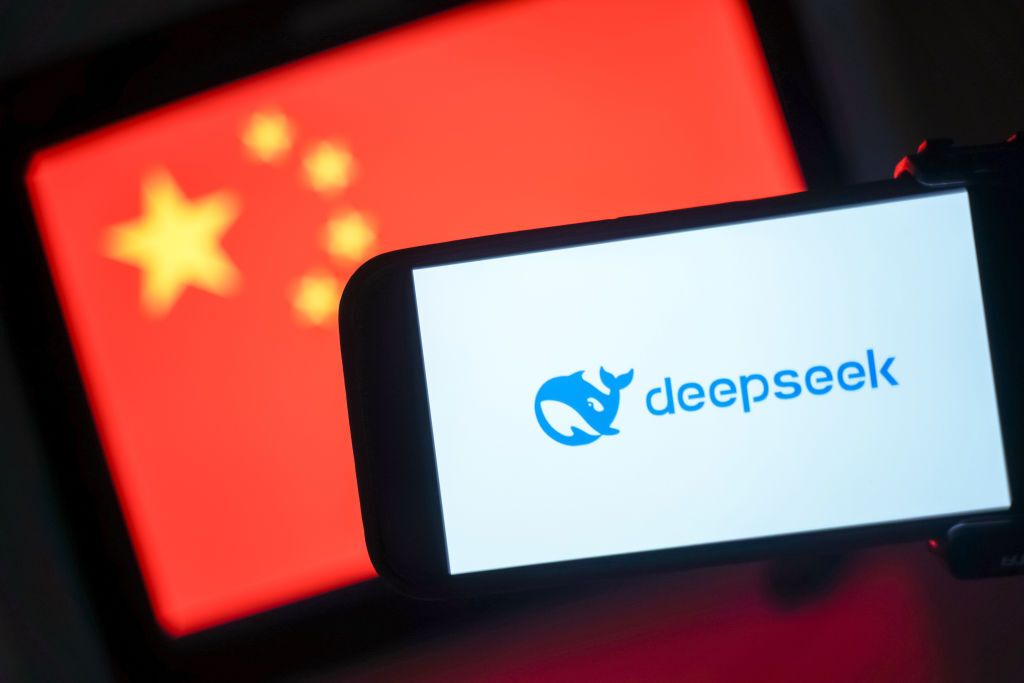
The Impact of DeepSeek-R1 on Web3-AI
DeepSeek-R1 introduces significant innovations that could reshape the landscape of AI, particularly within Web3 environments.
The Impact of DeepSeek-R1 on Web3-AI
Unlike most advancements in generative AI, the release of DeepSeek-R1 carries real implications and intriguing opportunities for Web3-AI. Recently, the artificial intelligence (AI) field was shaken by the introduction of DeepSeek-R1, an open-source reasoning model that performs at a level comparable to leading foundation models while being developed with an impressively low training budget and novel post-training methods.
DeepSeek-R1 challenges the commonly held beliefs about the scaling laws of foundation models, traditionally favoring extensive training budgets, particularly in the competitive domain of reasoning.
The open-weights feature of the model made it accessible to the AI community, leading to a swift replication of the model. Furthermore, DeepSeek-R1 significantly impacts the ongoing AI rivalry between China and the United States, highlighting the superior quality and innovative capabilities of Chinese AI models.
Understanding DeepSeek-R1
DeepSeek-R1’s innovations stem from incremental advancements in a well-established pretraining framework for foundation models. Its development involved three critical phases:
- Pretraining: The model is initially trained to predict the subsequent word using vast amounts of unlabeled data.
- Supervised Fine-Tuning (SFT): This stage focuses on optimizing the model for instruction-following and question-answering.
- Human Preference Alignment: A final tuning phase aligns the model’s outputs with human expectations.
Unlike many foundation models developed by the likes of OpenAI and Google, which follow a similar process, DeepSeek-R1 leveraged the existing base model from its predecessor, DeepSeek-v3-base, which contains a remarkable 617 billion parameters.
Key Innovations
DeepSeek-R1 produced not just one but two models, with the R1-Zero playing a significant role as an intermediate model specialized in reasoning tasks. This model utilized reinforcement learning largely, with minimal labeled data, showcasing the potential to develop state-of-the-art reasoning capabilities purely through reinforcement learning.
On the other hand, DeepSeek-R1 emerged as a more general-purpose model, fine-tuned to excel in reasoning tasks, building upon the methodologies introduced by R1-Zero.
Opportunities for Web3-AI
DeepSeek-R1 highlights several avenues for synergies with Web3 architectures, especially in:
- Reinforcement Learning Fine-Tuning Networks: The development of reasoning models through reinforcement learning fits well with decentralized models where nodes can be rewarded for training contributions.
- Synthetic Dataset Generation: DeepSeek-R1’s emphasis on synthetic reasoning datasets reinforces its compatibility with a decentralized environment, allowing nodes to autonomously participate in dataset generation processes.
- Decentralized Inference: The emergence of smaller, distilled versions of DeepSeek-R1 enables practical inference capabilities in Web3 environments, making advanced reasoning accessible in decentralized contexts.
- Data Provenance: The reasoning traces generated could lead to a new model of internet governance focused on transparency and accountability.
In conclusion, the release of DeepSeek-R1 could signify a transformative moment in generative AI, facilitating a convergence between advanced AI models and Web3 principles, potentially redefining the future landscape of AI technology.